Michele Antonazzi
Department of Computer Science, University of Milan
Email: michele.antonazzi@unimi.it
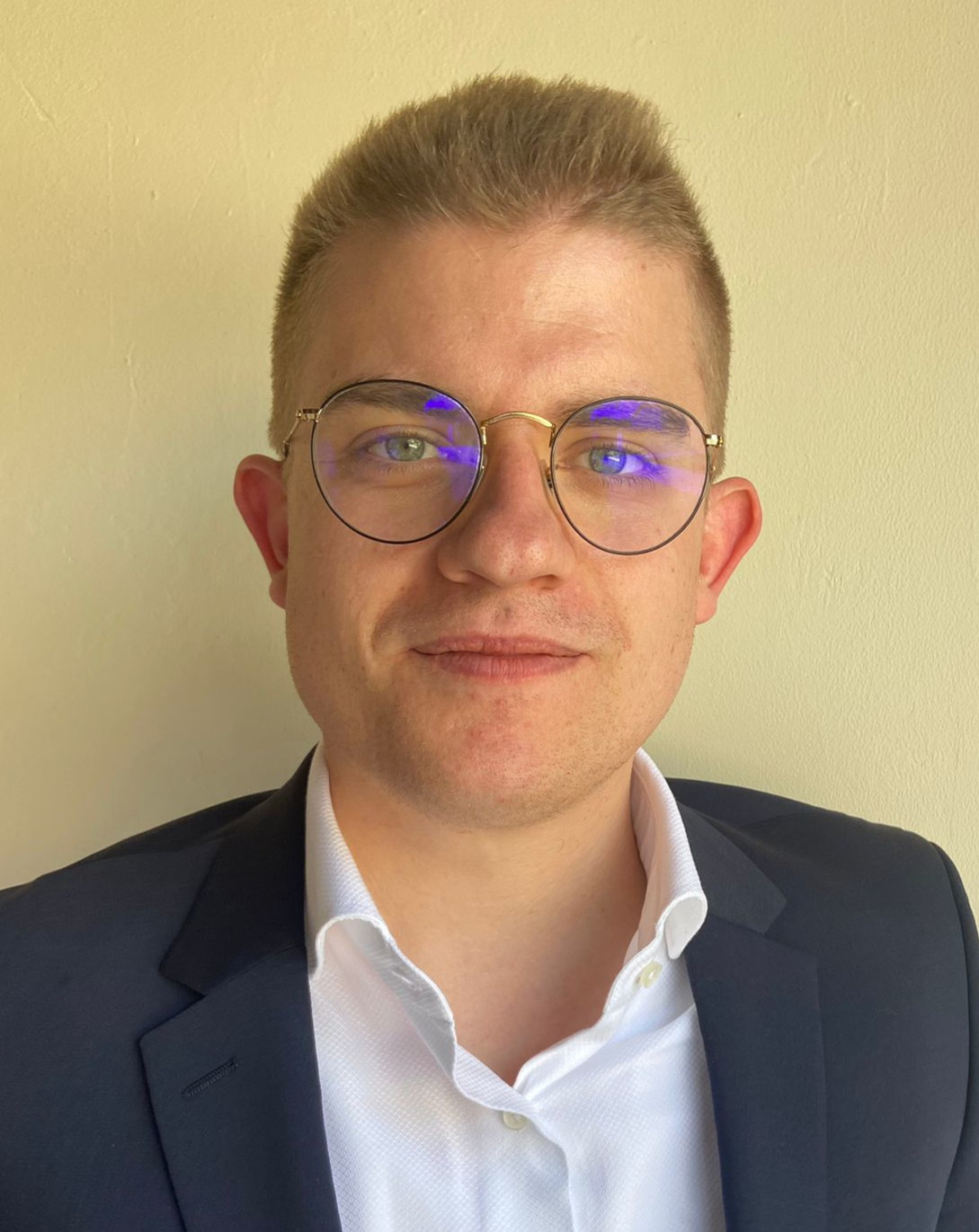
I’m PhD student at the Applied Intelligent Systems Laboratory (AISLab) at the University of Milan.
- Supervisor: Prof. Nicola Basilico
- Co-supervisor: Doc. Matteo Luperto
Research Topic: domain adaptation and privacy preservation for Robotic Vision.
The perception capabilities of indoor mobile robots are affected from domain shift when they are deployed in a new (and previously unseen) working environment. To overcome this, a robot should be able to qualify the vision modules to its operational context. This task is particularly challenging when the perception is distributed over the cloud to overcome the computational constraints of mobile robots. My research investigates novel methodologies for domain adaptation to address the scalability and privacy requirements of cloud robotics scenarios.